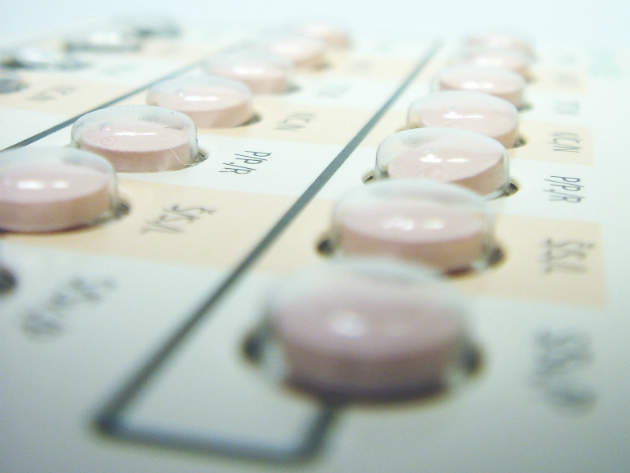
A comprehensive map of all existing drugs and how they work, created from information from several huge datasets, could be used to kick-start the next wave of drug discovery. Not only could scientists use the map to suggest promising new targets for diseases as diverse as cancer, mental illness and inflammatory conditions, it could also provide invaluable insight into where existing drugs can be used to treat different diseases.
Jointly led by scientists at the Institute of Cancer Research (ICR) in London, which also funded the study, the research brought together vast amounts of information from datasets including the canSAR database at the ICR, the ChEMBL database from the European Bioinformatics Institute (EMBL-EBI) in Cambridge, and the University of New Mexico’s DrugCentral database to create a map of all 1,578 licensed drugs and their mechanisms of action.
Key discoveries included that only 3.5% of the 20,000 human proteins have had drugs developed against them and that as many as 70% of all targeted drugs created so far work by acting on just four families of proteins – leaving vast swathes of human biology untouched by drug discovery programmes.
Co-leader of the study and head of data science at the ICR, Dr Bissan Al-Lazikani, explains how this first-of-its-kind map could help tackle one of the biggest challenges facing the drug discovery field: how do you innovate without taking risks that make the whole system unviable?
Elly Earls: Why did you decide that a comprehensive map of all existing drugs and their mechanisms of action was needed for the drug discovery community?
Dr Bissan Al-Lazikani: It’s really interesting actually – although modern medicines have existed for a long time, exactly how many of them work at a molecular level isn’t quite as well understood as you might imagine. But in order to innovate in drug discovery, you’ve really got to understand how successful drugs work and, when they’re less successful, what’s driving their lack of success. That allows you to be smarter about your next generation of drugs. You learn from the things that did work and try to extrapolate from that to expand into new areas of biology and drug discovery.
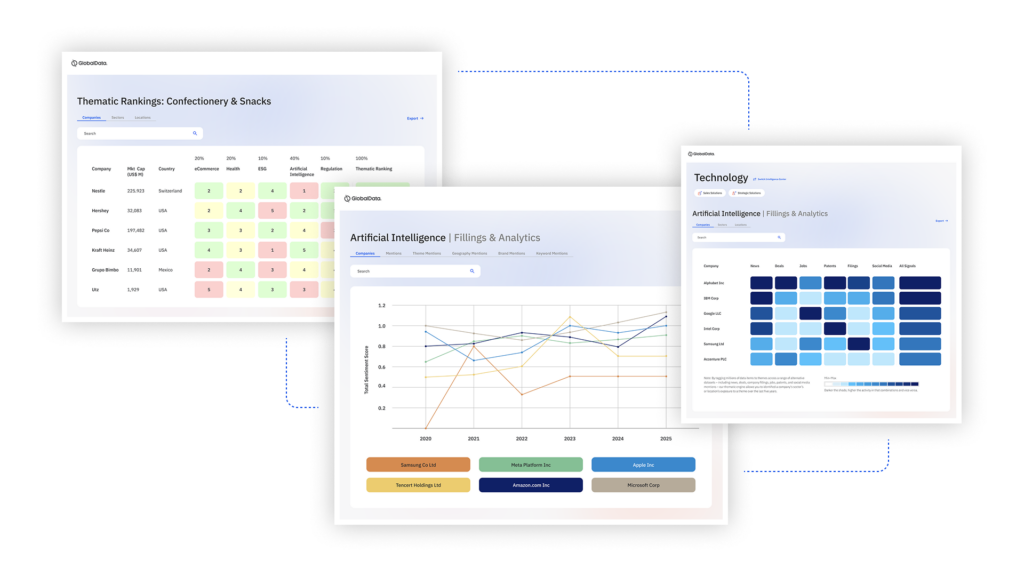
US Tariffs are shifting - will you react or anticipate?
Don’t let policy changes catch you off guard. Stay proactive with real-time data and expert analysis.
By GlobalDataSo ten years ago, myself and one of the co-authors of this study published an analysis that looked at targeted drugs and tried to really analyse how they work in the body and how they cause their therapeutic effect. This is an analysis of that same field, ten years on, but with a more sophisticated view, trying to understand how these mechanisms are mapping across different disease areas.
EE: How did you put the map together? It must have been quite a task!
BA: Yes, it was. The first study required a huge meta-analysis of a large body of literature – for example, of drug databases and the prescribing information that is supplied upon drug approval. Ten years on we also included all the drugs that had been discovered and approved over the last ten years. As you can see from the author list, there were quite a number of people involved across a multi-institutional collaboration. So again we compiled together a large number of databases, did a comprehensive meta-analysis of the literature and used the expertise that the three main groups involved in the study brought together, including my own expertise in cancer.
EE: What were the most interesting findings of the latest study?
BA: One of the interesting findings was that we’re still discovering drugs against very similar types of protein classes as we were 10 years ago. So there are an increasing number of me-too drugs, where you’re not innovating in terms of the biology, but you’re discovering new drugs that have better properties or are more efficacious, or have fewer side effects or work in areas where, for example, resistance may have arisen.
On top of this – and what’s even more interesting to me as a cancer scientist – is that we’ve found that oncology seems to be by far leading the way in terms of innovation. It’s where we’ve discovered the majority of ‘first in class’ drugs, the real rule breakers.
Another interesting thing we discovered was that there is an awful lot to be said for not necessarily just drugging the gene that’s causing the disease, but also trying to look for other genes or proteins around it that may themselves not be responsible for the disease but will impact the disease. This opens up a much wider pharmacological space for us to explore than just the exact disease drivers.
In cancer we see this happening quite a lot, where we’re not only drugging the cancer drivers but also other genes and proteins that are contributing to or supporting the disease. They’re not necessarily causing it but they are genuine vulnerabilities.
EE: What are the biggest challenges facing the drug discovery field at present and how will your comprehensive map help overcome these?
BA: The innovation gap remains the thing that is really a problem for us. What we know is that the more risks you have, the more likely you are to fail. The question is how do you innovate without taking such unbelievable risks that the whole system becomes unviable? This was one of the main drivers of why we did the analysis 10 years ago and why we needed to update it this time around – because by understanding how the current mechanisms work, you can start moving into novel areas of biology that maybe have characteristics that are similar to current successes.
What we’ve done is use the data we’ve gathered over years to develop predictive models for our target selection in drug discovery. So you build machine learning models that learn the characteristics of these successful targets and then try to identify completely novel targets that could be totally biologically unrelated but have characteristics that load the odds in their favour in terms of being successful drug targets.
We’ve published this work and will provide it for free to the drug discovery world – through the canSAR database – to help us in this innovation while managing risk. The next layer will be how to optimally link these innovative targets with the right patient population in order to ensure you are designing the best trial that would give you the answer you need to make a decision on the drug.