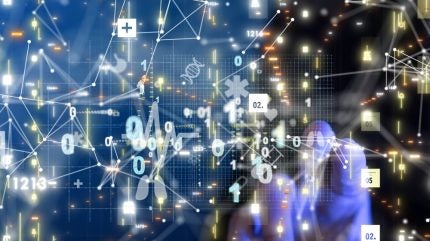
Artificial Intelligence (AI) has the potential to transform healthcare research and development. It has already begun revolutionizing the drug discovery process by streamlining research, potentially reducing time and costs required to bring new drugs to market. AI also shows great promise in medical diagnostics, speeding up image analysis and improving disease diagnosis.
One strategy – precision and personalized medicine (PPM) – stratifies patients based on their genomic and phenotypic variations to find targeted therapies. This approach has revolutionized disease treatment paradigms and patient outcomes, particularly in rare genetic diseases and cancers. According to GlobalData, PPM therapies are projected to be worth $106 billion by 2029, a rise of 44% since 2022[i]. However, for AI to develop accurate models, it requires access to extensive, high-quality patient data from diverse populations.
At the same time, the healthcare industry is struggling to make use of the vast amounts of data held in electronic health records (EHRs), and other medical sources, mainly due to the way in which this data is collected and stored. EHR systems were originally designed to manage comprehensive patient medical profiles, including medication histories, allergies, immunization status and laboratory results. The ultimate goal, with interoperability, is to facilitate data sharing among the patient’s healthcare providers, allowing for better care coordination.
Unfortunately, despite the vast amounts of healthcare data generated, much of it is trapped in silos and lacks integration. This fragmentation is primarily due to decentralized institutions employing non-standardized methods for data collection. Plus, a significant portion of healthcare data remains unstructured, such as free text notes and non-digitized images, which complicates data analysis and integration. This lack of harmonization prevents healthcare providers from leveraging data effectively, while researchers and life sciences organizations may find the data to be inaccessible.
This data is valuable, containing crucial information on medical histories, diversity and treatment success or failures. It presents an opportunity for biopharma researchers looking for new treatments and life science organizations looking for new markets. How can we bridge this information gap and consolidate big data sets effectively?
Who uses real-world data from EHRs?
Biopharma researchers:
In addition to billing and claims data, researchers are also often looking for clinical insights from nationally representative patient cohorts. Researchers require real-world evidence (RWE) for diverse patient populations for a wide variety of purposes. Beyond structured data, EHR data also often contains unstructured, or semi-structured data, that can be unlocked using AI and machine learning.
Government agencies:
Government agencies such as the FDA, are looking for information to see if treatments work, are safe, and what the current unmet need may be. Real-world data (RWD) from EHRs can be invaluable for this.
Commercial biopharma teams:
Data-driven commercial strategies can improve market access and support product launches. RWD from EHRs can demonstrate how therapies perform in the real world and it can help them target the right physicians and patients at the right time.
How do you transform real-world evidence into usable data?
Using AI and machine learning, useful real-world evidence can be extracted from EHR data, even from physician notes and patient charts. One company that is marking its mark in this area is US based Veradigm, which is an integrated data systems and services company that combines data-driven clinical insights with actionable tools, to help healthcare stakeholders improve the quality and efficiency of healthcare delivery.
Veradigm Network EHR Data is purpose-built for research, providing streamlined access to over 152m patient records[ii] by combining multiple EHR data sources. Using proprietary natural language processing (NLP) models, meaningful elements are extracted from unstructured and semi-structured data, providing deeper insights about the patient cohort of interest. Veradigm improves the integrity of the data using standardized organization and definitions of variables across sources.
Veradigm’s data sets incorporate data from a broad spectrum of medical practices, covering major specialties and family medicine, providing a comprehensive view of patient care and health outcomes across many different settings. Veradigm also has the ability to conduct both prospective and retrospective research, patient surveys and perform data clarification, due to its direct relationship with healthcare providers.
Using data responsibly
The transformation of EHR data through AI holds the potential to revolutionize healthcare by improving data management, enhancing patient care, and optimizing operational efficiency. However, the sharing and use of healthcare data can raise privacy concerns, so working with a company like Veradigm who adhere to data-sharing regulations and methods that preserve privacy is essential.
Veradigm is embracing AI in healthcare, recognizing its potential to revolutionize the industry. Through collaborations, research initiatives and innovation, Veradigm is positioned to develop solutions that have the potential to improve patient care, operational efficiency, and health outcomes.
For more information on how Veradigm can help with your real-world data, download the free paper below.
[i] GlobalData: Analyst Briefing: Precision and Personalized Medicine Is Rapidly Expanding Beyond Oncology, July 2023.
[ii] Figures provided by Veradigm, 2025.