The pharmaceutical industry is under increasing pressure, with rising research and development (R&D) costs, patent expiries, greater competition and pricing challenges all eroding profit margins. Pharmaceutical companies must ensure that they invest in the right areas of drug development to maximise profitability and minimise risk.
Artificial intelligence (AI) and its Machine Learning (ML) subset are increasingly being used across the entire pharma industry value chain, including for faster, more efficient and cost-effective drug discovery and repurposing as well as enhanced clinical trial design and recruitment.
Founded in 2013, Toronto-based biotechnology company Cyclica specialises in AI and data-driven drug discovery. It launched its deep learning platform, Matchmaker, in 2018. The platform allows for proteome-wide evaluation to determine complex drug polypharmacology in real time, meaning it predicts the on-target and off-target interactions that these compounds can have in vivo.
Traditional in silico approaches to drug screening tend to focus on a single protein target paradigm (potency), but compounds will actually hit multiple targets in the body. This can lead to off-target effects such as efficacy and toxicity issues, which could cause these drugs to fail early preclinical or clinical testing.
Matchmaker has been trained on millions of drug/target interactions, as well as thousands of protein 3D structures, and can screen across the human proteome in less than one second using vast amounts of biochemical and structural data. Cyclica’s Pareto-optimal embedded modelling (POEM) is a parameter-free, supervised ML algorithm that builds robust and reliable absorption, distribution, metabolism, excretion and toxicity (ADMET) models about molecules.
Powered by Matchmaker and POEM, Cyclica’s two integrated platforms, Ligand Design and Ligand Express, are used to accelerate the drug discovery process. Ligand Design performs a rapid, large-scale exploration of the vast chemical space and applies multiple selective pressures to design novel compounds that meet a partner’s requirements. By interrogating target interactions of drug candidates, Ligand Express reveals their mechanism of action and potential disease targets as well as off-target interactions, allowing for lead candidates to be prioritised.
How well do you really know your competitors?
Access the most comprehensive Company Profiles on the market, powered by GlobalData. Save hours of research. Gain competitive edge.
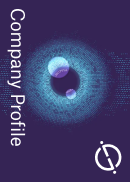
Thank you!
Your download email will arrive shortly
Not ready to buy yet? Download a free sample
We are confident about the unique quality of our Company Profiles. However, we want you to make the most beneficial decision for your business, so we offer a free sample that you can download by submitting the below form
By GlobalDataLigand Express also facilitates drug repurposing. In January, Cyclica reported that in a recent collaboration with AstraZeneca, MatchMaker and Ligand Express had outperformed mass spectrometry-based approaches for correctly identifying off-target drug interactions for small molecules, at a fraction of the time and cost.
Cyclica has partnered with over 100 global pharma and biotech companies and academia across many therapy areas such as respiratory, oncology, neurodegenerative diseases, infectious disease, ophthalmology and rare disease. According to the company, these partnerships have resulted in 64% of programmes resulting in actionable hits.
Cyclica’s future vision is to further expand its AI initiatives to become the forefront of innovation in drug discovery and be able to reduce drug discovery timelines from seven years down to two years, in order to get the best medications into patients’ hands faster.
Related Company Profiles
AstraZeneca Plc