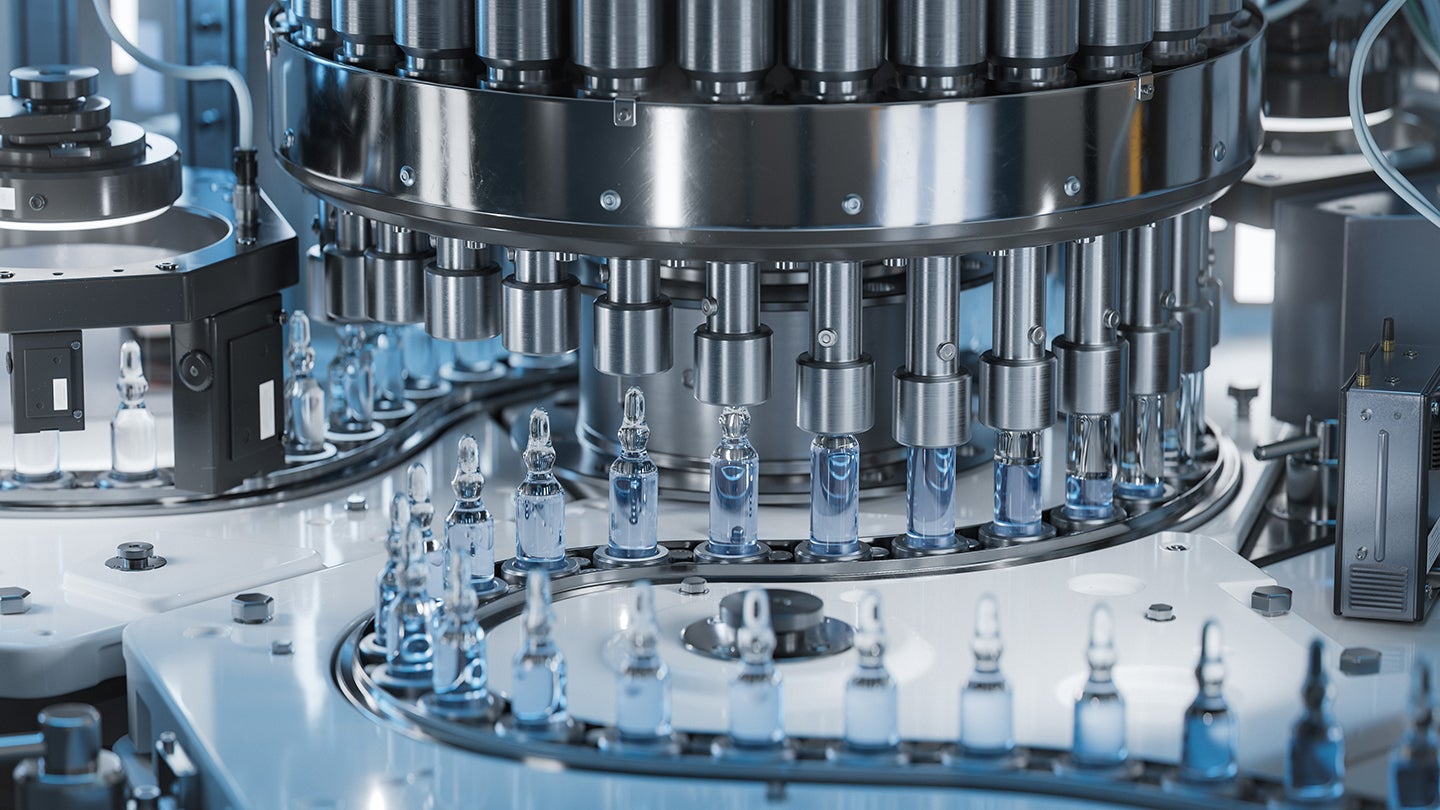
Maddy Irwin joined GlobalData’s Thematic Intelligence team in September 2022. She is particularly interested in analysing how tech themes affect the healthcare industry. Â
Lara Virrey: What are the most exciting developments in AI for the pharmaceutical industry today?
Maddy Irwin: AI can significantly accelerate drug discovery and development. Training AI on the vast amounts of genomic data, health records, medical imaging, and other patient information has allowed an increased understanding of the biological mechanisms of diseases. AI-assisted mapping of disease pathways and protein and drug interactions through specialist vendors has created a deeper understanding of targets and assisted in identifying novel proteins and genes that can be targeted to counteract them.
AI can also be used to predict three-dimensional (3D) structures of targets and accelerate the design of appropriate drugs that bind to them, through protein structure databases such as DeepMind’s AlphaFold. Once a set of promising lead drug compounds have been identified, AI can then assist in candidate drug prioritisation, ranking molecules for further assessment.
Aside from identifying drug targets, AI has also been used successfully in the virtual screening of compounds, such as identifying those that can bind to ‘undruggable’ targets, de novo drug design, drug repurposing, and identification of treatment response biomarkers. AI algorithms have shown the potential to condense a typical four or five-year exploratory research phase into less than a year, significantly reducing the time and cost to get a drug to market, which is particularly important for indications with few treatment options.
Lara Virrey: How can companies in the pharmaceutical sector benefit from advances in generative AI in particular?
Maddy Irwin: In drug discovery, AI can analyse vast amounts of data to detect patterns and trends and assess the biological performances of drug candidates to find the best for clinical testing. Generative AI, for example, can predict novel drug candidates or molecular structures based on patterns and trends found in data.
See Also:
Generative AI can also be used to design novel chemical compounds. For example, researchers at the University of Toronto have developed an AI system that can create proteins not found in nature. The system uses the same technology as OpenAI’s Dall-E image creation platform. In the future, this could assist in discovering new drug candidates with unique properties, with the potential to create treatments for diseases that currently have no effective options.
How well do you really know your competitors?
Access the most comprehensive Company Profiles on the market, powered by GlobalData. Save hours of research. Gain competitive edge.
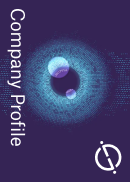
Thank you!
Your download email will arrive shortly
Not ready to buy yet? Download a free sample
We are confident about the unique quality of our Company Profiles. However, we want you to make the most beneficial decision for your business, so we offer a free sample that you can download by submitting the below form
By GlobalDataGenerative AI may also assist in improving communication and streamlining in pharma manufacturing. ChatGPT could create optimisation plans, predictive maintenance schedules, and detect anomalies in available information.
Lara Virrey: What are the barriers to implementation of AI in the pharmaceutical industry?
Maddy Irwin: While AI has shown the potential to transform drug discovery processes, it faces many challenges. These include the quality and appropriateness of data, continued assurance of drug safety and efficacy, educating the scientific community to increase buy-in, and issues around intellectual property rights.
The use of generative AI in healthcare also raises concerns about protecting patient privacy and sensitive medical data, including using patient data to train generative AI algorithms. Further, generative AI increases cybersecurity risks and the potential for misuse or unauthorised access to healthcare data. Existing strict regulatory regimes around data privacy in general and healthcare specifically will need to be updated to reflect advancements in AI before generative AI can be trusted in healthcare.
Lara Virrey: Which companies are the leading adopters of AI technologies in the pharmaceutical sector?
Leading adopters of AI technologies in the pharmaceutical sector include IQVIA, Pfizer, Sanofi, Novartis, AstraZeneca, Johnson & Johnson, and Bristol Myers Squibb.
For example, Bristol Myers Squibb is leading the use of AI for drug discovery and development. In July 2020, BMS collaborated with ReviveMed to use its AI platform to better understand mechanisms of response and resistance to immunotherapies in patients with cancer.
Another collaboration was in October 2020 with the ML-driven drug discovery and development company Insitro. The five-year collaboration focuses on discovering and developing novel therapies for treating rare neurological diseases, amyotrophic lateral sclerosis, and frontotemporal dementia.
The same year, BMS collaborated with Sensyne Health to apply ML for rare blood disease research and disease progression within the broader group of myeloproliferative neoplasms. In June 2022, BMS partnered with Owkin to use its AI platform to enhance clinical trial design and execution for cardiovascular drug trials.
In March 2023, BMS also partnered with Viz.ai to create a new tool called Viz HCM, designed to spot signs of hypertrophic cardiomyopathy. The tool aims to flag patients showing potential signs of the disease, so they can undergo further review and diagnostic testing.