
Drug manufacturers and tech companies are investing heavily in artificial intelligence (AI) in the hope it will drastically reduce the cost and time involved in developing new pharmaceuticals. AI also has the potential to enable more efficient, targeted drug delivery, thus improving patient outcomes.
Along with tech giants such as Microsoft, BenevolentAI and the private-public consortium ATOM, at least 76 start-ups using AI in drug discovery have launched in the past two years, including CytoReason.
Founded in 2016 and based in Tel Aviv, Israel, CytoReason generates innovative biological insights through its AI model of the immune system. From new cancer immunotherapies to autoimmune and neurodegenerative research, the company combines immunology domain expertise with AI technology to accelerate breakthroughs hidden in the massive amounts of data.
“Leveraging our platform and proprietary data, we offer our pharma partners an unparalleled resource, becoming their system immunology sounding board,” says David Harel, CytoReason co-founder and president. “CytoReason’s proprietary machine-learning engine enhances its performance with each new dataset introduced. Our platform enables innovative big-data analysis, focusing on solving mysteries in immune related disorders and therapies, affecting the cost of target identification, reducing the risk of clinical trials, and bringing the world closer to an affordable personalised medicine.”
AI and biomarkers
CytoReason recently announced that its AI model had been used to discover novel biomarkers to one of the most common anti-inflammatory (anti-TNF) drugs, Remicade. The research was carried out in collaboration with Israel’s Professor Shen-Orr from the Technion and Professor Chowers from Rambam Hospital, using lesion RNA data, combined with CytoReason’s data model.
In the first public demonstration of its technology, the company used three different publicly available and independent clinical trials to study the RNA from lesion tissues, identifying patients that responded to the drug, versus those who did not. Specific biomarkers in blood and tissue samples were discovered, which predicted when anti-inflammatory drugs such as Remicade will be effective.
How well do you really know your competitors?
Access the most comprehensive Company Profiles on the market, powered by GlobalData. Save hours of research. Gain competitive edge.
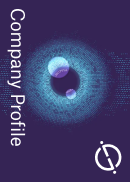
Thank you!
Your download email will arrive shortly
Not ready to buy yet? Download a free sample
We are confident about the unique quality of our Company Profiles. However, we want you to make the most beneficial decision for your business, so we offer a free sample that you can download by submitting the below form
By GlobalDataIn general, anti-TNF or TNF inhibitor drugs have excellent therapeutic efficacy with minimal side effects, making them world leaders in treating autoimmune disorders (Humira, Remicade and Enbrel sales in 2017 amounted to approximately $30bn). However, despite these advances, up to 30% of patients do not respond to the therapy and up to 46% lose response over time, says Harel. “Until now, the only way to know whether the patient was a responder or non-responder was trial-error, treating the patient for three months while waiting for the outcome,” he explains.
“Personalising the treatment, identifying which patient will respond to which drug, has significant impact on all stakeholders. Patients receive the correct treatment faster, the costs of trial-and-error are slashed, and the workload of the health system is reduced.”
Predicting responses to anti-TNF drugs
CytoReason’s AI model uses proprietary and public data to create a set of immune-signalling maps. Each map is a model of the immune system and specific to the disease studied, the type of tissue sampled and the chosen treatment, e.g. an inflammatory bowel disease, gut biopsy and treatment with Remicade. The maps include all the relevant genes, proteins and cells (often the entire human genome).
“We used data from several clinical trials studying gene expression from gut biopsies before patients were treated with Remicade,” says Harel. “Using our model, we were able to discover which genes came from which cells, allowing us to recreate the map for each patient. Comparing the maps of those who responded to those who didn’t, we saw that patients with high level of certain cells in the gut lesion were less likely to respond to Remicade.”
Moreover, CytroReason was able to determine which groups of genes were associated with each other, flagging up the most influential genes and proteins. Since the maps are tissue specific, a comparison of the gut map and the blood map easily identified influencing genes and proteins that can be found in both the gut lesion and blood flow.
Challenges and benefits
The complexity of this area of study remains one of the key challenges in immune research. The cells are the main actors in a complex system, communicating with one another, sending and receiving certain proteins, coordinating the immune efforts. The most common measurement technologies measure the genes and the proteins in bulk, breaking the cells into their molecular components.
CytoReason addressed this challenge by using an AI model that ‘learned’ which proteins came from which cells, making the reconstruction of the cellular network a simple task.
“In addition, the model integrates the measurements from dozens of different measurement technologies, on gene, protein, and cell levels,” explains Harel. “CytoReason is the only healthcare AI company to develop a specific cell-based model for the immune system, with an extensive database.”
The company’s partners and customers include global pharmaceutical giants and research organisations that use these maps to discover new drug targets.
“They expand the use of existing drugs, predict side effects and identify patients who are likely to respond,” says Harel. “This makes clinical trials safer for patients, shorter in duration, and more predictable and focused for the pharmaceutical company. Using an AI model, CytoReason has developed a map of the immune system that sheds light on biological interactions that until recently were considered ‘too complicated to understand’.”
AI and the future of pharma
Personalised medicine has been the focal point of all pharma and healthcare stakeholders for decades. Its promise of improving outcomes and reducing costs started taking shape in the 1990s and has been rolled out to patients with varying degrees of success ever since.
Future development will take us in this direction, providing holistic answers to increasingly specific questions, at the fingertips of the physician and the patient.
“The introduction of big-data technologies allows personalised medicine to take a new, exciting, direction,” Harel concludes. “It allows for higher resolution models to simulate complete heterogeneous tissues and all in real-time. This is exactly where CytoReason aims to be, while focusing on the most impactful system in the human body – the immune system.”