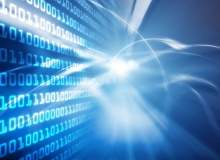
Over the past few decades, the clinical data management process has steadily been upgraded from a labour-intensive paper-based system to a more efficient software-driven model of shepherding clinical trial information through the drug development cycle.
But despite all the technological advances of the 21st century, effectively collecting, storing and analysing complex clinical data is a task which still presents immense challenges to even the largest pharmaceutical companies and research organisations.
The potential for automated data management lies just over the horizon, but many organisations that have not committed to the implementation of fundamental data management standards risk not being able to take advantage of such advanced systems. Furthermore, as standards such as those of the CDISC (Clinical Data Interchange Standards Consortium) come closer to being made compulsory by regulatory authorities like the US Food and Drug Administration (FDA), the need to embrace standards-driven clinical data practices is becoming more urgent by the day.
Octagon Research Solutions is a US-based clinical data specialist that advises the pharma industry on creating a standards-led data management process from initial design to final submission. We sat down for a round-table discussion with Octagon’s chief information officer David Evans and senior director of clinical data strategies Barry Cohen to get some insight on the clinical data landscape, as well as the challenges and benefits of creating a big-picture strategy for data management.
Chris Lo: What does end-to end data management actually mean?
David Evans: We term it the clinical information lifecycle. We look at information that goes from the design stage, meaning the design that goes into a protocol, all the way through to submission of that information to a regulatory authority. Through discrete stages over that lifecycle, it is designed, collected, processed and stored in some fashion; it’s then analysed and reported on, before being compiled and submitted to an agency who review it for an approval. So those stages together constitute the lifecycle of clinical data in our industry. Each one of those areas has its own data management practices that go on, depending on what role they’re playing.
How well do you really know your competitors?
Access the most comprehensive Company Profiles on the market, powered by GlobalData. Save hours of research. Gain competitive edge.
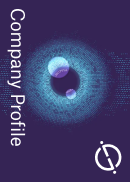
Thank you!
Your download email will arrive shortly
Not ready to buy yet? Download a free sample
We are confident about the unique quality of our Company Profiles. However, we want you to make the most beneficial decision for your business, so we offer a free sample that you can download by submitting the below form
By GlobalDataBarry Cohen: I think there’s general agreement in the industry today that to be more standards-based in each stage of the lifecycle is to be not only regulatory compliant, but also more process-efficient. The key to process efficiency, and this is our key when we work with organisations, is to find a way that they can be consistent in their use of standards across all of their studies, not only some of them.
CL: What problems do pharma companies or research organisations commonly have when it comes to data management?
DE: How they are functionally organised presents a huge challenge. You have data management organisations and statistical, programming organisations that are separated culturally or organisationally, which don’t talk to each other. Nor do they use the same standards, or the same language in some cases. So it’s not a technological thing, it’s a cultural, organisational, sometimes political thing.
BC: If the organisation is intending to do a filing in the US and they don’t have a plan for how they’re going to incorporate the use of CDISC standards, they are definitely going to have a roadblock. All the guidance from the FDA is pointing towards the intent to make these standards mandatory within a couple of years from now. If they’re not already on the path to being CDISC standards-based, then they’re not going to be able to make a regulatory-compliant filing.
DE: There was a draft guidance that came out in February from the FDA that talked about study data and submission of that information to the FDA. One of the points in there was that the FDA is requesting that there is a study date plan in place at the IND [investigational new drug] stage, meaning the earliest filing of that protocol. So that’s the movement of the FDA to step into the standards world earlier and earlier in the process, not just at the time of submission.
CL: How important is metadata, or ‘data about data’, to the whole data management process?
DE: This idea of metadata – or the characteristics associated with groups of data objects – has not been matured to the point where it should be in this industry. What we’ve been describing is the ‘what’ of the data object, and now we need to know the who, the why, the where, the how – other questions associated with data, so we have a true understanding of how to trace that particular piece of data throughout this lifecycle.
In this industry, if we want to look into automating this practice, being able to automatically validate this information or automatically generate analysis and tables and listings, we must have a much richer set of context for the data than we currently do. If we look at other industries, Amazon is a good example of these data object-oriented approaches that have taken hold and led to great strides in the automation of the process. Here in pharmaceutical research, we’re easily a decade behind in how these technologies can be applied, and I believe this is because standards have not been put in place.
CL: How can software systems help to keep track of clinical data?
DE: The industry has been growing up with point solutions. So the technology providers come in and provide a particular technology for one point in the lifecycle. EDC [electronic data capture] systems are a good example of that; they’re just at the collection part of the process. So we have this rich EDC industry that is a technology built for one purpose only.
How do we then move that information from that point solution into another system that would do the analysis, or the reporting of that information, carrying with it its context? So this emergence of common standards, common understanding of those data items, becomes necessary.
BC: The idea behind automated trial design, and this is definitely a technology that the industry is beginning to move towards now, is that it would allow the user to create, and then provide in a machine-readable form, all the metadata that’s describing all the activities that are going to happen during the trial.
These would include data collection, so the metadata needed to drive processing in an EDC environment. But it wouldn’t end there, because it would also have the metadata that describes the data in storage in a clinical data warehouse, and the analysis that would occur on that data once it was warehoused. Finally, the metadata to describe the data as it would be submitted to the FDA.
There needs to be a vehicle or a system that’s going to make that data available to all points in the life cycle. The current systems are point solutions at each stage of the life cycle, and they’re not designed to provide this metadata to every point. This brings us to a second key piece of technology, and that’s the metadata repository, or metadata registry. This is an environment in which all of that metadata that’s describing all the activities across the lifecycle is stored in one central location, and made available from there to all these different stages of the lifecycle.
CL: How does this kind of metadata-driven technology fit in with new ideas about adaptive trial design?
DE: It’s very interesting you bring that up, because without the production of this machine-readable metadata, if you don’t have this approach of decomposing everything into these machine-readable objects, it’s very difficult to do the adaptive part of it because you’re not able to automate the changing of the trial, or the workflow associated with that change, without great difficulty. So while the concept of adaptive trial design has been around for the better part of five years, the technology has not been there to enable it as efficiently as possible. All that metadata needs to be tracked automatically, such as in a metadata registry, in order for the regulatory authorities to be comfortable that the traceability of that information is intact.
CL: So you see data management automation as an enabler for the more complex trial design ideas?
DE: It certainly has to be that way. You have to have the foundation of the data management processing and the capture of the information in a standard fashion, along with the richness of that data and metadata, to then enable a use-case that is much more complex, like automated trial design or adaptive trials. Each one of those use-cases is predicated on that foundation of machine-readable information in a data management system.
From my standpoint, this is an exciting time. We’ve reached a point in our industry where automation is finally going to become a topic for implementation, because of the use of standards in the industry. That becomes the enabler for these foundations, which then enable the more complex systems.