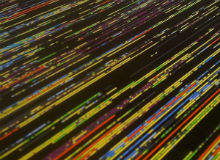
The amount of information that can be accessed at the click of a button today is staggering, and its potential if it were to be fully exploited, even more so. This is particularly true in the field of biotechnology, where the discovery of an important link between two pieces of research that may previously have gone unnoticed could vastly speed up the drug discovery process, ultimately saving lives.
The problem, of course, lies in the impossibility of ever having enough manpower to manually sift through the ballooning amount of data pouring into all sectors of the global economy on a second-by-second basis, let alone make any sense of it.
Enter text mining. A discipline that uses advanced computational techniques and machine learning to trawl reams of biomedical and clinical textual data and make those important links, it is fast becoming an indispensable part of the scientific research process and, despite challenges surrounding the availability of data, is set to become more sophisticated.
Information explosion
Facebook users share 1.3 million pieces of content on the site every minute of every day, while global scientific output has been found by bibliometric analysts at the Swiss Federal Institute of Technology in Zurich to double roughly every nine years.
Moreover, a 2011 McKinsey Global Institute (MGI) report on ‘Big Data’ predicted not only that the amount of information and data across all sectors of the global economy was expected to increase at an annual rate of 40%, but also that the exploitation of this vast resource could generate significant economic benefits.
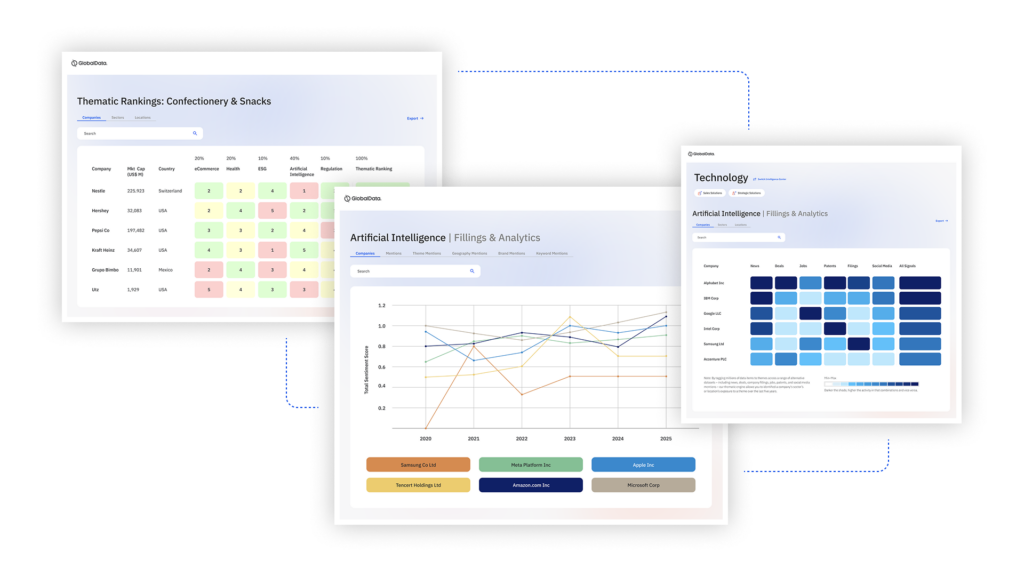
US Tariffs are shifting - will you react or anticipate?
Don’t let policy changes catch you off guard. Stay proactive with real-time data and expert analysis.
By GlobalDataFor example, the report found that effective and creative use of large data sets by the US healthcare sector could generate more than $300bn in value per annum and reduce national healthcare expenditures by around 8%. Today, text mining is one technology that is being used to help make sense of this data deluge.
What is text mining?
In its simplest form, text mining, which has been around since the late 1990s in the biotechnology arena, but has significantly developed in sophistication over that time, involves analysing large collections of documents to make sense of the information contained within them. This information could correspond to concepts, relationships or patterns that would be extremely difficult, if not impossible, to discover manually, and that could help to answer existing research questions or lead to new avenues of research.
To discover this information, text mining involves the sequential application of different techniques from areas including: information retrieval (IR) (the most well-known IR system is Google); natural language processing (the analysis of human language so that computers can understand it as humans do), which includes information extraction (IE) (the process of obtaining snippets of information from unstructured documents automatically); and data mining (the process of identifying patterns from large sets of data).
These various stages of processing can then be combined to form different task-driven text mining workflows. Or as Professor Sophia Ananiadou, director of the National Centre for Text Mining (NaCTeM) at the Manchester Institute of Biotechnology, prefers to describe the process, it’s a bit like making a cake.
"You start with a very plain vanilla base, which is the unstructured text, then you start adding layers," she explains. "You add some cream, you add some strawberries, and that could be, for example, technical terms or named entities such as genes, proteins, diseases or symptoms. Then you add another layer, which is the relationships between them – protein X binds with gene Y, symptom X is indicative of disease Y, and so on. Next, it’s the discovery of what we call ‘events’, which combine simple relationships into more complex ‘nuggets’ of information, for example, one protein may bind with another only under specific environmental conditions, or a symptom may only be associated with a disease in certain parts of the population. And it gets more complex from there."
The key is that, because software tools rather than people are doing the analysis, the amount of time, and therefore money, which can be saved by researchers, is phenomenal. "With text mining, you can save up to 80% of your time, so it really is a technology that you cannot do without anymore," Ananiadou stresses.
Limitations for users
Earlier in the evolution of text mining technology, a key issue that was raised by researchers was the fact that different text mining tools – the tools created by NaCTeM, for example – were unable to talk to the tools created by other research institutions. But Ananiadou is happy to report that today, this issue has largely been resolved.
"Not only my team but other groups have addressed this issue by creating interoperable platforms to allow text mining tools from different groups to talk to each other," she says. "At NaCTeM, for example, we have created an interoperable text mining infrastructure which actually allows the creation of text mining workflows that use not only our tools, but also the best of breed of other people’s tools."
Today, the main challenge facing researchers is not related to text mining technology, but rather to the availability of data. "The main limitations we have are the publishers," Ananiadou remarks. "For example, there are copyright issues that mean we can’t apply our tools to all academic articles, and certain results can’t be shared. However, to allow our tools to work as accurately as possible, we have to use big data sets, so we really need to be able to have access to everything."
Things are improving on this score, however. The UK, for example, has introduced an exception to copyright law to permit the use of data analysis, including data and text mining, for non-commercial research purposes. Unfortunately, copyright law still presents a barrier to these disciplines across the rest of Europe.
Exciting times
Technology-wise, Ananiadou predicts exciting times as the sector moves forward. Not only are text mining tools set to improve in terms of performance and accuracy, the future potential for text mining as a part of the wider area of artificial intelligence (AI) systems will mark a new stage in the field’s development in the coming years.
"The future really lies in integrating text mining with other disciplines, such as robotics and chemoinformatics, so it will be used from more of an AI perspective," she predicts.
In the meantime, Ananiadou has plenty to keep her occupied at NaCTeM, and she cites some of her most interesting projects as: EMPATHY, in which text mining methodologies are being used to help develop metabolic pathway models; Big Mechanism, which aims to automate the process of intelligent, optimised drug discovery in cancer research with text mining tools; and Mining for Public Health, a project being carried out in conjunction with the National Institute for Health and Care Excellence (NICE) to transform the way evidence-based public health (EBPH) reviews are conducted.
"Text mining doesn’t necessarily give you all of the answers, but its potential to speed up the research process and suggest more promising paths for drug discovery is huge," Ananiadou concludes.