Scientists at the Institute of Cancer Research (ICR) in London recently published research that demonstrates the utility of artificial intelligence (AI) to stratify luminal-A breast cancer into five sub-types.
Published in Nature Partner Journals Breast Cancer, this research may allow clinicians to personalise treatments more effectively in the future.
Two pioneering studies of the early 2000s distinguished five major molecular subsets of breast cancer based on gene expression clustering.
These sub-types were discovered to be separated by the presence or absence of three important receptors: estrogen receptor (ER), progesterone receptor (PR), and the human epidermal growth factor 2 (HER2). This provided the basis for current treatments for breast cancer.
Breast cancer is a highly heterogeneous disease; thus, the treatment of breast cancer has become increasingly personalised to specific molecular signatures associated with the disease.
Researchers at the ICR aimed to provide a new level of personalisation for patients who suffer from the most common form of breast cancer, the luminal-A subtype.
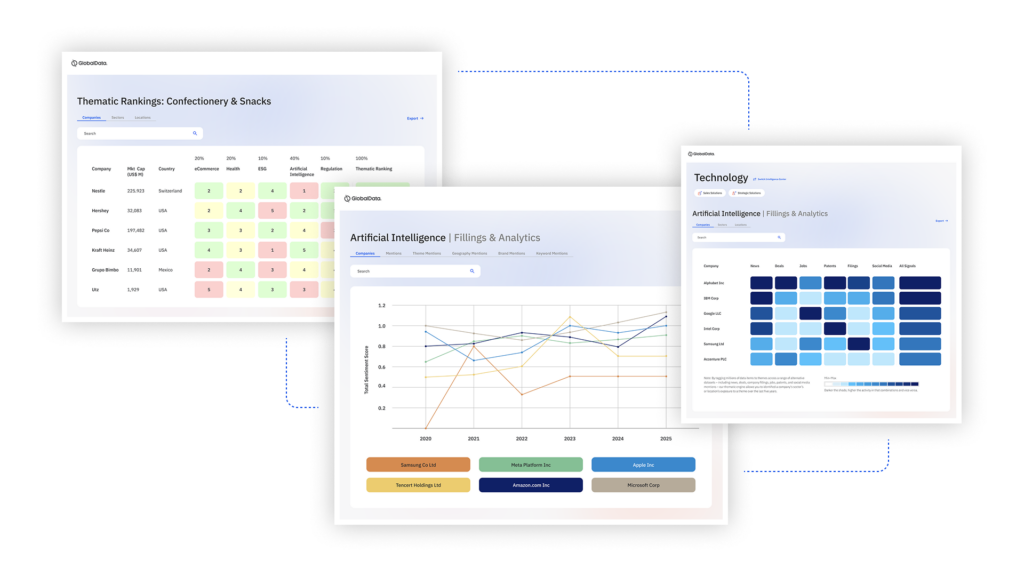
US Tariffs are shifting - will you react or anticipate?
Don’t let policy changes catch you off guard. Stay proactive with real-time data and expert analysis.
By GlobalDataApplication of AI
Applying AI and machine learning to gene sequences and molecular data from breast tumours, five distinct sub-types of patients with luminal-A breast cancer were identified in this study.
Critically, two sub-types that are more likely to respond to immunotherapy were identified due to presence of an immune signature used to predict sensitivity to checkpoint inhibitors in other cancer sub-types.
Considering the recent introduction of Roche’s Tecentriq (atezolizumab) into the treatment paradigm for triple-negative breast cancer, this has clear translational significance.
As Tecentriq + Celgene’s Abraxane (protein-bound paclitaxel) was approved in the US for patients based on a clear benefit for programmed death-ligand 1 (PD-L1) positive patients, this study may highlight patients who are most likely to benefit from Tecentriq and other checkpoint inhibitors within the luminal-A sub-type.
This study does not challenge the overall classification of breast cancer, but does find additional differences within the current sub-divisions of the disease that have important implications for treatment.
The study further highlights how AI and machine learning can be utilised to serve the healthcare community through analysing Big Data and informing personalised medicine and treatment decisions.
Such examples are not new in the field of breast cancer. For example, the startup Kheiron Medical, a UK business dedicated to utilising machine learning, is developing tools to support the work of breast radiologists to identify breast cancer earlier.
The lead ICR researcher said that we are at the “cusp of a revolution in healthcare”, and GlobalData expects further positive implementation of AI and machine learning in the next five to 10 years.