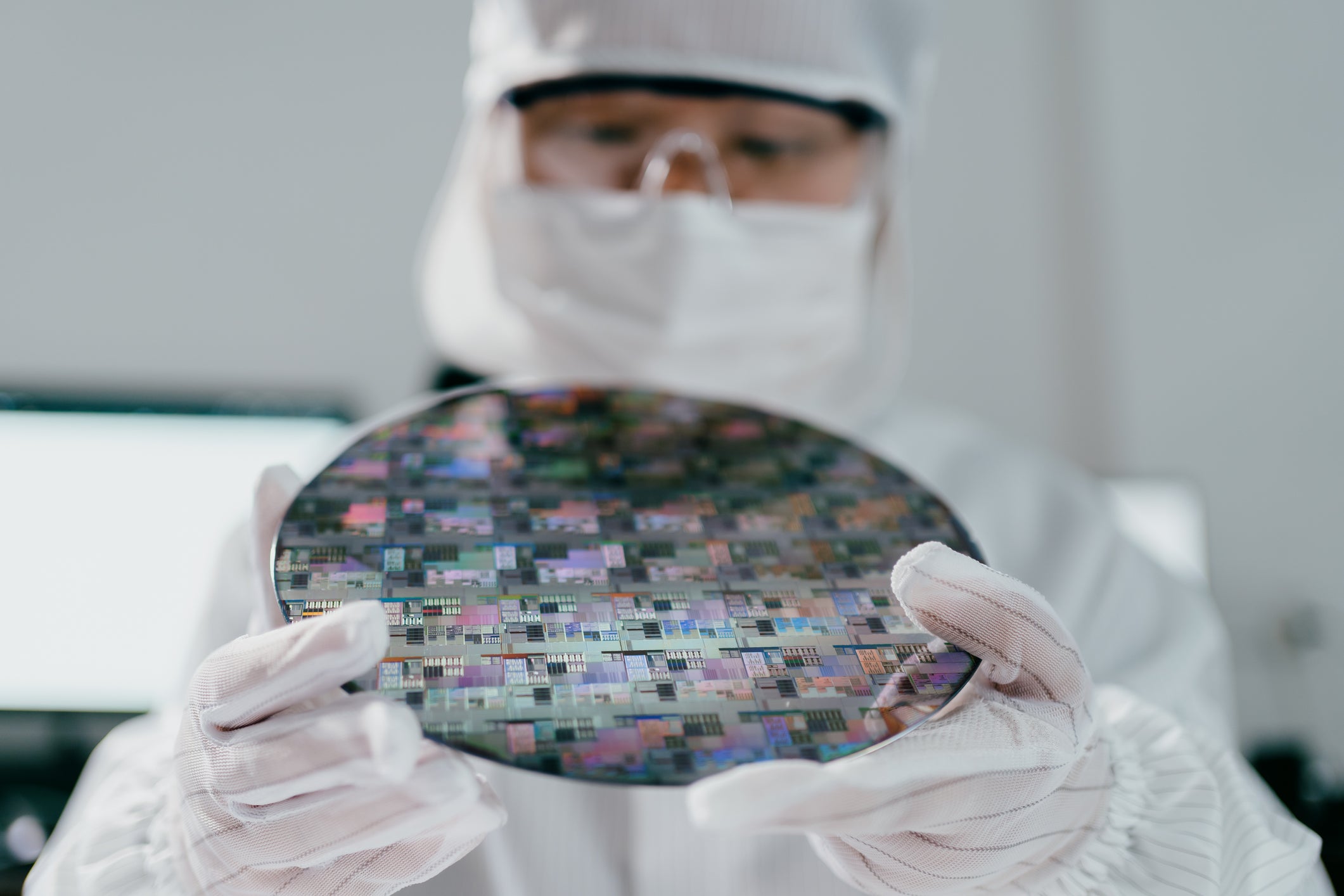
Chinese science and technology research institute, Damo Academy – a subsidiary of ecommerce giant Alibaba – has announced that its “Multi-Modality to Multi-Modality Multitask Mega-transformer” (M6) artificial intelligence (AI) system has increased its number of parameters from 1 trillion to 10 trillion, far exceeding the trillion-level models previously released by Google and Microsoft. According to the announcement, this makes M6 the world’s largest AI pre-training model.
According to the academy, M6 has achieved ultimate low carbon, high efficiency in AI models using 512 graphic processing units (GPU) to train a 10 trillion parameter neural network within ten days. Compared to the GPT-3, a large model released by the OpenAI research laboratory last year, M6 achieved the same parameter scale with only 1% of its energy consumption.
M6 is a general AI model developed by Damo Academy, with multi-modal and multi-task functions. According to the company, its cognitive and creative capabilities surpass most AI in use today, and it is especially good at design, writing and Q&A functions. The academy says that the model could be used widely across the fields of ecommerce, manufacturing, literature and arts, scientific research and more.
In the mainstream form of AI – machine learning (ML) – the usual structure is a “neural network” in which a layered array of probabilistic gates is set up. Unlike normal computing logic gates which will always respond in the same way, neural gates are more like neurons in a human brain – they may respond one way or another when triggered. As a neural network “learns”, the probabilities in each gate (the “weights”) are adjusted. When AI researchers describe the number of parameters in their systems, they typically mean the number of “weights” in it – and a saved set of mapped weights represents a “trained” AI which has been set up for a given task.
The new thing about M6 is that it has hundreds or thousands of times the number of “neurons” compared to other AI systems currently being trialled, perhaps enabling a learning ability that is more like the human brain. According to Alibaba, M6 has been applied in over 40 scenarios, with a daily parameter volume in the hundreds of millions.
“Next, we will deeply study the cognitive mechanism of the brain and strive to improve the cognitive ability of M6 to a level close to human beings. For example, by simulating human cross-modal knowledge extraction and understanding of humans, the underlying framework of general AI algorithms is constructed,” said Zhou Jingren, head of the data analytics and intelligence lab at Damo Academy.
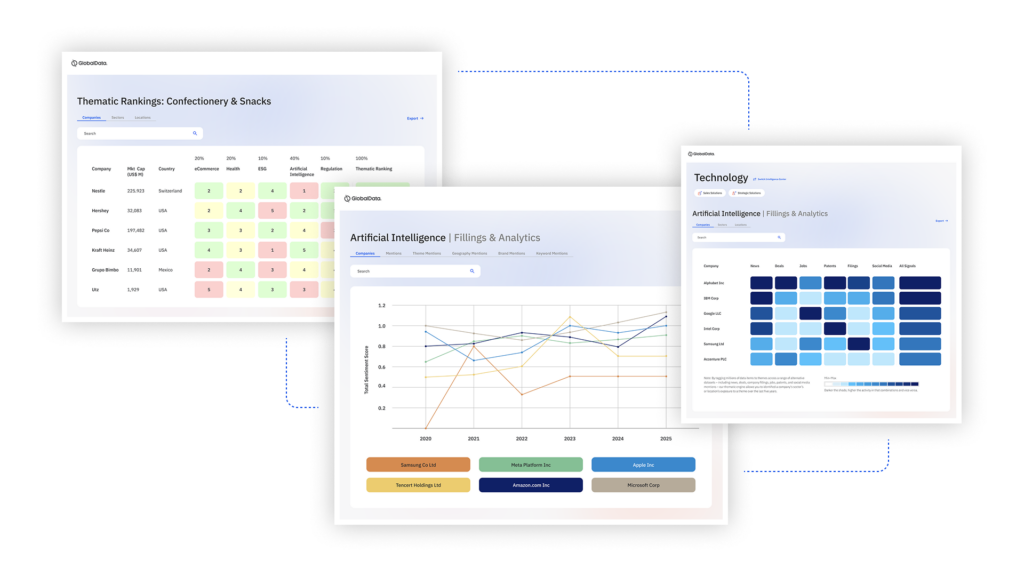
US Tariffs are shifting - will you react or anticipate?
Don’t let policy changes catch you off guard. Stay proactive with real-time data and expert analysis.
By GlobalData“The creativity of M6 in different scenarios is continuously enhanced to produce excellent application value.”
What is multi-modal AI?
Multi-modal AI is a new AI paradigm which combines various data types (images, text, sound, numerical data, etc.) with multiple intelligence processing algorithms to achieve higher and faster performances. By combining these data types, multi-modal AI might outperform modal AI in many real-world problems.
Multi-task learning in machine learning (ML) is a method in which multiple learning tasks are solved simultaneously while exploiting commonalities and differences across tasks.
When an AI model is created, it typically focuses on a core or central benchmark. A single model or an ensemble of models are primarily trained according to that benchmark. While generally speaking this can achieve acceptable performances, it ignores other information that could be helpful to improve the core metric. Multi-task ML shares representations between related tasks, enabling the model to perform more efficiently in relation to the original task.
China and its battle for AI supremacy
The Alibaba Damo Academy (Academy for Discovery, Adventure, Momentum and Outlook) is an academically-oriented hybrid research and development facility. It was established in 2017 in Hangzhou – where Alibaba is headquartered – and operates independently from its parent company.
The entity primarily focuses on scientific research and core technology development. It claims to have invested hundreds of billions of yuan in three years to develop core basic technology.
It aims to create a research ecosystem in China combining cutting-edge technologies (such as quantum technology), breakthroughs in core technologies (such as AI and chips) and the application of key technologies (such as databases).
Last month, Damo Academy announced a new chip design based on advanced 5-nanometre (nm) technology.
Fuelled by a global chip shortage and sanctions placed on companies restricting them from accessing the global semiconductor market, Chinese firms have been making headway towards self-sufficiency in producing high-end chips.
Tech giants such as Alibaba, Tencent and Huawei – which have all been affected by the ongoing US-China trade war – have made efforts to design their own chips in hopes of easing dependence on foreign suppliers.
However, the problem of manufacturing these chip designs remains. Currently, Taiwan Semiconductor Manufacturing Company (TSMC) and South Korea’s Samsung Electronics are the only two foundries in the world capable of mass-producing 5nm chips. Establishing such foundries is not simple: the necessary lithography machines are extremely expensive and mostly are not manufactured in China.
Nevertheless, China has in recent years established itself as a global player in AI-related research. In March, when the M6 model was first introduced, Jack Clark, former policy director of the OpenAI research laboratory, commented:
“The scale and design of these models are amazing. This looks like a manifestation of the gradual growth of many Chinese AI research organisations.”
Senior analyst at GlobalData, Michael Orme, called this development “another ‘Sputnik moment’ for the US on top of the hypersonic missile.”
Last month, the Pentagon’s former chief software officer, Nicolas Chaillan, warned that China was already winning the AI race due to the US military’s sluggish digital transformation, private actors’ reluctance to work with the state and an abundance of ethical debates stifling innovation.
“We have no competing fighting chance against China in 15 to 20 years. Right now, it’s already a done deal; it is already over in my opinion,” Chaillan told the Financial Times in October.
Indeed, Chinese President Xi Jinping has made it crystal clear that achieving global supremacy in technology areas, including AI, semiconductors, quantum computing, etc., is at the top of his agenda.
At an annual conference in June, Xi emphasised that China’s scientific and technological independence should be seen as a “strategic goal for national development.”
As Orme puts it, such moves are being made “in the context of what’s going on at the Chinese Communist Party’s third history congress”. The analyst quoted George Orwell: “‘who controls the past controls the future. Who controls the present controls the past.'”