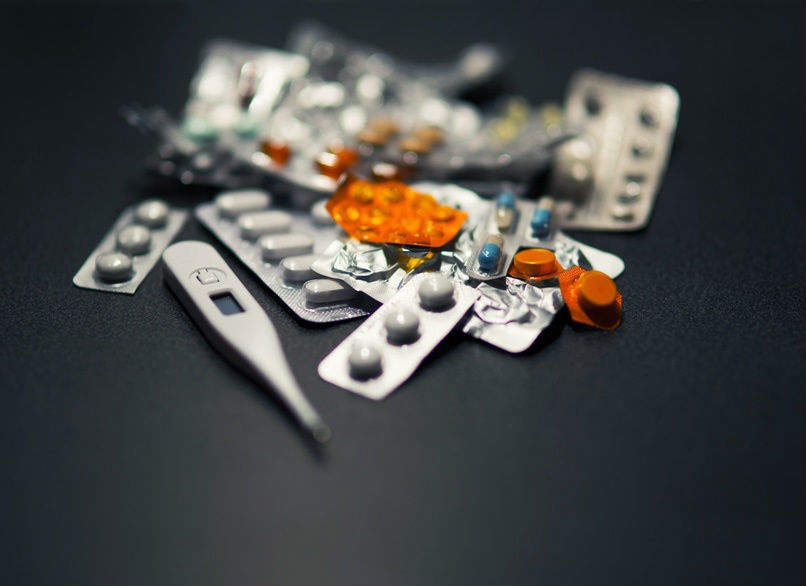
Some 100,000 people in the US die from prescription drug side effects every year and 7% of all hospital admissions in the country are due to adverse drug reactions, costing the healthcare system nearly $150bn. This statistic has inspired a group of scientists at the University of California (UC) to develop a model that could be used to predict a drug’s side effects on different patients. And while their research is still only at the proof of concept stage, its potential for reducing the number of people exposed to drugs that could be harmful to them is significant.
Although many side effects are anticipated and accepted by doctors and patients, particularly if medicines are being used to treat life-threatening conditions, adverse drug reactions place a significant burden on the country’s healthcare system, one that isn’t discussed often enough.
"The side effects of drugs cost one dollar for every three dollars of drug sales – it’s actually an astonishingly high figure and a big problem with delivery of healthcare," says Bernhard Palsson, Galetti professor of bioengineering at the Jacobs School of Engineering at UC San Diego (UCSD).
"It’s one of these inconvenient truths – the cost of side effects is much higher than one expects."
It’s this situation that has led Palsson, along with a team of researchers at UCSD, to use cutting-edge technology to develop a model that could be used to predict a drug’s side effects on different patients based on their genetic make-up. Their initial proof of concept study, published in October in the journal Cell Systems, is a big step forward for the field.
How well do you really know your competitors?
Access the most comprehensive Company Profiles on the market, powered by GlobalData. Save hours of research. Gain competitive edge.
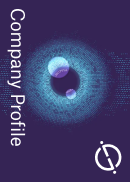
Thank you!
Your download email will arrive shortly
Not ready to buy yet? Download a free sample
We are confident about the unique quality of our Company Profiles. However, we want you to make the most beneficial decision for your business, so we offer a free sample that you can download by submitting the below form
By GlobalDataDifferent patients, different side effects
The modelling technique tested during the study works on the concept that, depending on our metabolic make-up, we react differently to drugs – some individuals experience side effects and others don’t. Specifically, it is able to predict how different people will respond to ribavirin, a drug used in conjunction with other drugs for hepatitis C, which causes anaemia – a condition characterised by a decrease in red blood cell levels – in 8-10% of patients.
Researchers used genomic and metabolomics data obtained from blood samples of 24 individuals to build personalised models that simulate how the drug will affect red blood cells. They then used these predictive models to understand, at the metabolic level, why some individuals experienced side effects to ribavirin while others did not.
"There are known genetic markers for whether or not you would be susceptible to the drug’s side effects or not and the genetic information we had for the study participants matched with what the model was predicting," says Aarash Bordbar, who did this research as a PhD student in Palsson’s systems biology research group.
Scaling up
The proof of concept study was a clear success, but with only 24 participants involved, there is still a lot more work to be done – requiring studies on hundreds of people rather than dozens – to prove that the model’s predictive capabilities hold up. And these studies are unlikely to be carried out using ribavirin, as it’s a drug that is currently being phased out and replaced by better treatments.
Instead, the team is on the lookout for drugs that are likely to have side effects not only in red blood cells but also in platelets, cells which can be destroyed by certain drugs, affecting how well the blood clots. "We would then do a proof of concept study like the one we’ve already done for a series of other drugs and once we have found a commonly prescribed therapeutic in a group of test compounds that is likely to have a big healthcare effect, we would scale up from there," Palsson explains.
One candidate currently being considered is Tolcapone, a medicine used to treat Parkinson’s disease. "It’s a drug that, depending on the genetic make-up of the patient, may inadvertently bind to protein in the red blood cell, whereas the main target is the brain," Palsson explains.
Paradigm shift
Ultimately, the researchers hope to play a key role in the paradigm shift already underway in the pharmaceutical industry towards personalised medicine. "The traditional approach is that we’re looking for a particular protein in the body and we’re going to hit it with one drug and it will be a magic bullet. But over the last 15 to 20 years, a lot of technology – like DNA sequencing – has been developed so we’re now able to understand what the systemic effect of a drug is," Bordbar explains. "My interest is trying to tease out those effects at the cellular, systemic scale and hopefully increase drug safety and efficacy."
"The fact that we can start to implement that on an individual basis, or on a personalised basis, based on the genetic sequence of the recipient is really a transformational idea in the field," Palsson adds.
Specifically, the researchers hope there will be two key applications for their modelling technique. Firstly, it could be used to build up a database over time, which would then be used by physicians to decide, based on the genotype of a patient, whether to prescribe a particular drug to them or not. "This would shield vulnerable patients from nasty side effects based on their genotype," Palsson notes.
And secondly, there is potential to deploy it during drug development, helping pharmaceutical companies to stay away from compounds in the drug development pipeline that are likely to have side effects in a lot of patients. "We’ve had a few high-profile cases where drugs are withdrawn from the market post-approval because of side effects, which is an extraordinarily costly event for a company," Palsson remarks. "Having a predictive tool during the development of the drug would be extremely useful."
Drug side effects may not be widely discussed but the statistics speak for themselves. Palsson concludes: "Before we got into this study, we had no idea how serious drug side effects are broadly speaking in the industry and how costly they are – there is a real need for being able to do what we’re trying to do."