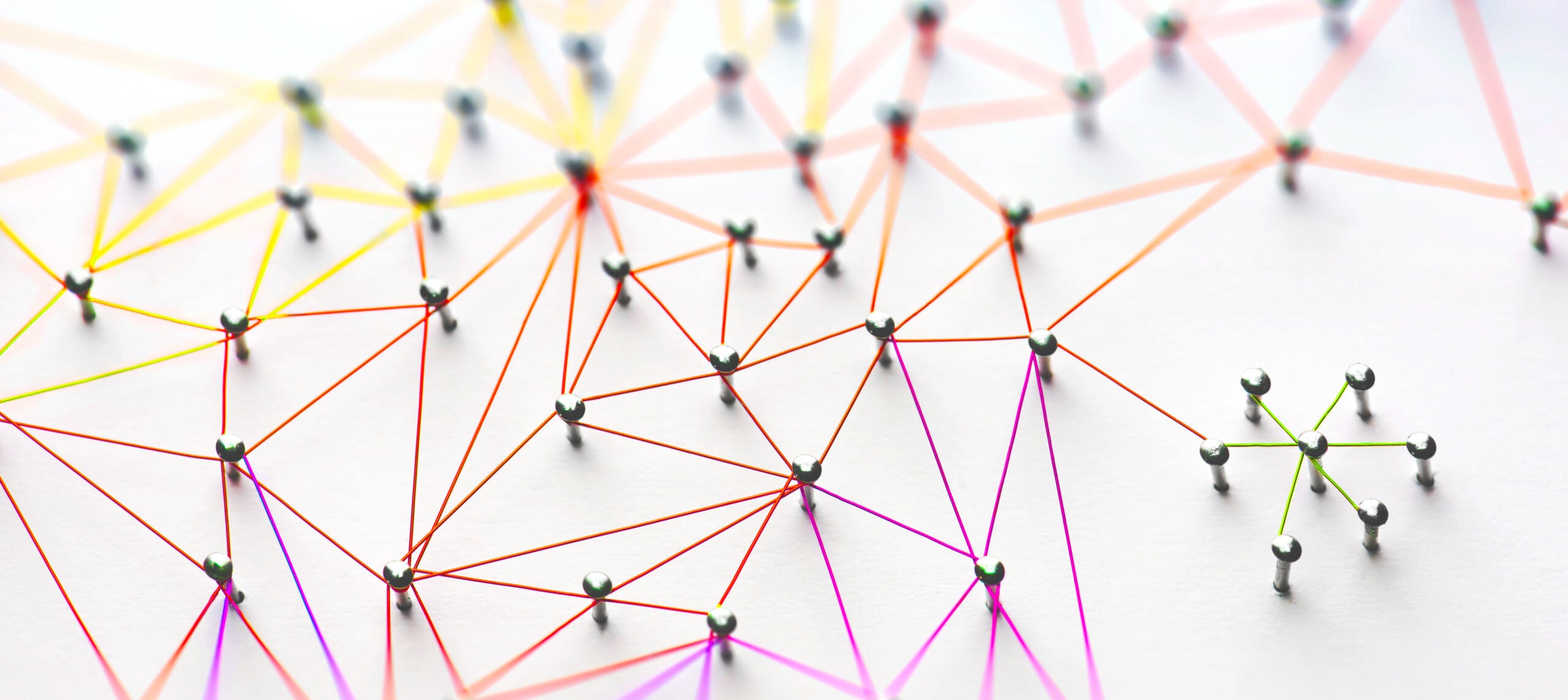
Since the publication of the human genome in the 2000s, scientists have increasingly studied how individual molecules interact with each other inside cells. The interactions between different molecules such as proteins or RNA are described by the term gene regulatory network (GRN).
These connections are governed by proteins called gene transcription factors, which control which genes are expressed and which are silenced. “The cell has an internal logic that has been honed by 3.7 billion years of evolution,” says Dr. Andrea Califano, professor of medicine at Columbia University’s Institute for Cancer Genetics in New York City. Several molecular interactions take place between proteins, DNA, and RNA, and scientists are now using technology to accurately reconstruct the logic of these interactions, he says. This process has been described as GRN inference.
Mapping these interactions can translate into the study of drugs and their impact on the network, explains John Quackenbush, PhD, professor of computational biology and bioinformatics at Harvard University, Boston. While such predictions need to be clinically confirmed, these algorithmic approaches are now being applied to develop and repurpose drugs. Scientists have taken to developing new tools and using such methods in recently initiated clinical studies.
Repurposing drugs using GRN inference
GRN inference could not only quickly estimate which existing drugs could impact a disease, but could also set the parameters for the creation of novel drugs. The idea is that mathematical modeling can be used to deduce the potential effect of a drug on targeted molecules, says Paola Lecca, PhD, assistant Professor at the Smart Data Factory Laboratory, Faculty of Computer Science, Free University of Bozen-Bolzano in Italy.
Network inference is a starting point, as it could deduce what effect different drugs would have on a disease even if they are not designed for it, explains Lecca. Running these tests on a large number of drugs electronically is not only faster but also cheaper, says Jorge Goncalves, PhD, professor at University of Luxembourg’s Centre for Systems Biomedicine.
See Also:
In February, Quackenbush and colleagues released their tool called EGRET. It is part of what Quackenbush calls his “network zoo”, which features different GRN inference software named after creatures. He expects the team to release another tool named after the mythological phoenix before the end of the year.
How well do you really know your competitors?
Access the most comprehensive Company Profiles on the market, powered by GlobalData. Save hours of research. Gain competitive edge.
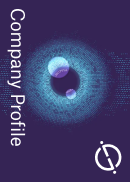
Thank you!
Your download email will arrive shortly
Not ready to buy yet? Download a free sample
We are confident about the unique quality of our Company Profiles. However, we want you to make the most beneficial decision for your business, so we offer a free sample that you can download by submitting the below form
By GlobalDataThese tools are crucial in translating increasing amounts of complex data into research with a tangible clinical impact. The balance between overly complex datasets and the need for valuable information remains a challenge for the field as it edges towards clinical use, he adds.
Califano and his lab at Columbia University have started using GRN inference to repurpose cancer drugs. In a collaboration with the Columbia University Medical Centre, Califano’s lab is using its GRN inference approach in n-of-1 clinical trials for 130 patients with different cancers, like bladder cancer, colorectal cancer, or glioblastoma.
In this collaboration, Califano’s team first uses data from The Cancer Genome Atlas Program to build models of each cancer. These models detect the master regulators responsible for cancer transcription and tumor maintenance. The regulators are then crosschecked with tumor samples extracted from each patient, which are sequenced for their DNA and RNA.
Subsequently, about 350 oncology and non-oncology drugs that are either FDA approved or in Phase II and Phase III clinical trials are then tested at low concentrations to study the drugs’ mechanisms of action and effect in cell lines that match the tumor samples, explains Califano. Through this, he says, “we essentially rediscover [the drug’s] pharmacology in the context of the specific cells where we want to make predictions”. The potential effects of those drugs are then predicted and cross-tested on the different tumors, he adds.
These processes are part of Darwin OncoTarget/OncoTreat, the proprietary technology of the company DarwinHealth, which Califano co-founded. In addition to creating wider GRN inference tools, his lab also looks at the biology of cancer systems.
This approach is also being tested in an ongoing umbrella trial for pancreatic cancer patients called HIPPOCRATES. Here, patients are enrolled, and their tumor samples are used to select potential treatments using Darwin Health’s technology. While this trial has only enrolled only six patients so far, Califano says that the team has already seen responses in mice transplanted with their tumors. According to Torbjörn Nordling, PhD, assistant professor at the Department of Mechanical Engineering at the National Cheng Kung University in Tainan, Taiwan, showing and understanding a drug’s mechanism of action on a deeper level is one of the promises of GRN inference in drug development.
Nordling says that this would be especially valuable in developing new treatments for cancer where mutations in the genome play a key role. Califano describes cancer as a disease of transcription factors. Examining the mechanisms through a GRN approach could also make these drugs more precise, says Nordling. Califano adds that GRN inference can be considered for other conditions like Alzheimer’s disease.
At the moment, Califano says that academia leads the way in developing these approaches and getting them to a proof-of-concept stage. However, there are already signs of businesses using network inference to develop treatments like the US-based company GNS Healthcare, says Quackenbush. In May 2021, DarwinHealth announced its research collaboration with Bristol Myers Squibb titled the Novel Cancer Target Initiative, which uses systems biology to develop new drug targets, as per a May 2021 press release.
Balancing complexity is a challenge for clinical use
Although the research community is finding new ways of combining further data, the issue now is in finding the right data, says Quackenbush. There is a need to reduce the actual complexity of the input data while making relevant predictions, he says. At the same time, there sometimes is not enough data from the lab, says Lecca, which can result in suboptimal inference modeling. Goncalves says this will be a continuous challenge for the field, stating that models can only tell us what is in the data. Making assumptions going beyond that could lead to bias, he adds.
Despite the recent research spurt, GRN inference is mostly in the stage of exploratory science where groups are building their models, explains Quackenbush. The question now is how these tools can be translated into being clinically used, he says.
“Once we move into the clinic, we actually need to be able to reduce [the complexity of] this exploratory model into something clinically useful, and that requires that we select measurements and features that are interpretable to a physician,” explains Quackenbush. These models are made of different mathematical equations and algorithms, which should be hidden in their original form and managed by user-friendly interface, to make sure these tools are easy to use, Lecca adds.
This is referred to as a feature selection problem, a phenomenon also seen in other areas like statistics, says Nordling. It essentially means that those who are developing the GRN inference models need to select a small but sufficient set of features that will map the network without burdening it with unnecessary details.
The field still needs to focus on these aspects before it can be put to clinical use, says Quackenbush. This will likely include the need for creating more reliable algorithms and tools, which can be replicated in the clinic, he adds.